Why Machine Learning Is the Future of Data Analysis
Discover why machine learning is revolutionizing data analysis. Explore its benefits, applications, and how ai/ml development services empower businesses with predictive insights and smarter decision-making.

Data analysis is undergoing a transformative revolution, driven largely by advances in machine learning (ML). In an era where data is often described as the “new oil,” extracting meaningful insights from vast and complex datasets has become a cornerstone of modern decision-making. Machine learning—a subset of artificial intelligence (AI)—has emerged as a game-changing tool, enabling analysts to move beyond traditional methods and harness the full potential of their data. Moreover, businesses are increasingly seeking AI/ML development services to implement cutting-edge solutions tailored to their needs. This article explores why machine learning is poised to dominate the future of data analysis, examining its capabilities, applications, and transformative impact on industries worldwide.
The Evolution of Data Analysis
Traditionally, data analysis relied on statistical models and human-driven exploration. Analysts would use tools like Excel or SQL to parse through data, manually identifying patterns, correlations, and trends. While these methods have been effective, they often struggle to keep up with the velocity, volume, and variety of modern data streams.
Enter machine learning. Unlike traditional statistical models, ML algorithms can automatically learn from data and improve their performance over time. They require minimal human intervention and are capable of analyzing vast amounts of information at unprecedented speeds. This shift marks a significant departure from the static and often manual techniques of the past, opening the door to dynamic and predictive insights.
What Makes Machine Learning Superior?
1. Scalability and Speed
Machine learning models excel at processing large-scale datasets. With the exponential growth of big data, traditional methods often become cumbersome and inefficient. ML algorithms, however, thrive in these environments, leveraging parallel processing and advanced computation to handle terabytes of data in minutes.
2. Pattern Recognition
Humans are adept at spotting patterns in small datasets, but ML algorithms can detect complex, non-linear relationships that would be nearly impossible for the human brain to identify. For example, neural networks can uncover subtle interactions in data, such as correlations between seemingly unrelated variables.
3. Automation
One of the most transformative aspects of machine learning is its ability to automate repetitive tasks. From data cleaning to feature selection, ML algorithms streamline the analysis process, freeing up analysts to focus on strategic decision-making.
4. Predictive Capabilities
Traditional analytics are often descriptive, focusing on “what happened” in the past. Machine learning, by contrast, excels at prediction, enabling businesses to answer “what will happen” and take proactive measures. Whether it’s forecasting sales or predicting equipment failures, ML turns data into actionable foresight.
5. Adaptability
Unlike static models, machine learning algorithms are dynamic and adaptable. They learn from new data and continuously refine their outputs, ensuring their predictions remain accurate even as underlying conditions change.
Applications of Machine Learning in Data Analysis
1. Business Intelligence (BI)
Modern BI tools, such as Tableau and Power BI, increasingly integrate machine learning capabilities to enhance their functionality. ML models can automatically detect anomalies in sales data, predict customer churn, and even suggest strategies to optimize revenue.
2. Healthcare
Machine learning is revolutionizing healthcare data analysis by enabling predictive diagnostics and personalized medicine. For instance, ML algorithms can analyze patient records to predict the likelihood of disease outbreaks, recommend tailored treatments, and identify at-risk individuals.
3. Finance
In finance, machine learning is used for fraud detection, algorithmic trading, and credit risk assessment. By analyzing transaction patterns, ML models can flag suspicious activities in real time, helping institutions mitigate risks and protect assets.
4. Retail and E-commerce
From recommendation engines to inventory management, machine learning is reshaping retail. By analyzing customer behavior, ML algorithms can personalize shopping experiences, forecast demand, and optimize supply chains for maximum efficiency.
5. Manufacturing
Predictive maintenance powered by machine learning is helping manufacturers reduce downtime and extend equipment life. ML models analyze sensor data to identify potential failures before they occur, saving costs and improving productivity.
6. Marketing
Marketers are leveraging machine learning to analyze consumer sentiment, segment audiences, and optimize campaigns. Tools like natural language processing (NLP) enable brands to extract insights from social media, reviews, and other unstructured data sources.
7. Scientific Research
In fields like genomics, astronomy, and climate science, machine learning is accelerating discoveries by analyzing massive datasets that would otherwise be unmanageable. For example, ML algorithms are used to identify exoplanets, model protein structures, and predict climate trends.
Benefits of Machine Learning in Data Analysis
1. Improved Accuracy
By reducing human error and incorporating sophisticated algorithms, machine learning significantly enhances the accuracy of data analysis. This is particularly crucial in fields like medicine and finance, where small errors can have significant consequences.
2. Cost Efficiency
Automating data analysis tasks reduces labor costs and accelerates processes. Over time, the efficiency gains from ML can translate into substantial financial savings for organizations.
3. Unbiased Insights
Machine learning algorithms rely on data-driven processes, minimizing the risk of bias that can arise from human judgment. While algorithmic bias is still a concern, it is more manageable than human subjectivity in many cases.
4. Enhanced Decision-Making
With real-time insights and predictive capabilities, machine learning empowers organizations to make informed decisions quickly. This agility can be a critical competitive advantage in fast-paced industries.
Challenges and Limitations
While machine learning offers immense potential, it is not without challenges.
1. Data Quality
ML algorithms are only as good as the data they are trained on. Poor-quality data, missing values, or biased datasets can lead to inaccurate predictions and flawed insights.
2. Interpretability
Many ML models, particularly deep learning algorithms, are often considered “black boxes,” making it difficult to understand how decisions are made. This lack of transparency can hinder adoption in sensitive applications like healthcare or law.
3. Resource Intensity
Training and deploying machine learning models require significant computational power and expertise. Organizations must invest in infrastructure and skilled professionals to fully leverage ML’s potential.
4. Ethical Concerns
Machine learning raises ethical questions around data privacy, surveillance, and algorithmic bias. Ensuring ethical practices and regulatory compliance is essential for building trust in ML-powered systems.
The Road Ahead: Why Machine Learning Is the Future
1. Integration with Artificial Intelligence
As machine learning continues to evolve, its integration with broader AI technologies like natural language processing, computer vision, and robotics will unlock new possibilities. For instance, AI-powered virtual assistants can analyze customer interactions in real-time, providing personalized support at scale.
2. Democratization of Machine Learning
With the rise of no-code and low-code platforms, machine learning is becoming accessible to non-experts. Tools like Google AutoML and Microsoft Azure ML enable businesses of all sizes to implement ML solutions without requiring in-depth technical knowledge.
3. Edge Computing and IoT
The proliferation of Internet of Things (IoT) devices is generating massive amounts of real-time data. Machine learning models deployed on edge devices can analyze this data locally, enabling faster decision-making and reducing latency.
4. Industry-Specific Innovations
Machine learning is driving innovation across industries, from autonomous vehicles in transportation to precision agriculture in farming. These sector-specific applications underscore ML’s versatility and transformative potential.
5. Continuous Learning and Adaptation
The ability of ML algorithms to learn and adapt over time ensures their relevance in an ever-changing landscape. As more data becomes available, these models will only become more accurate and valuable.
Conclusion
Machine learning is undeniably reshaping the field of data analysis, offering unparalleled capabilities in processing, interpreting, and acting on data. By automating complex tasks, uncovering hidden patterns, and delivering predictive insights, ML empowers organizations to make smarter decisions faster. As a result, the demand for AI/ML Development Company is growing rapidly, as businesses seek to integrate these cutting-edge technologies into their workflows. While challenges remain, the continued advancement of machine learning technologies promises a future where data-driven insights are not just a competitive advantage but a fundamental aspect of success.
As industries embrace machine learning, the role of data analysts will also evolve. Rather than manually sifting through datasets, analysts will increasingly focus on strategy, creativity, and ethical considerations. In this new paradigm, machine learning is not just a tool—it is the engine driving the future of data analysis.
What's Your Reaction?


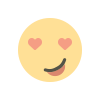



