What Are the Limitations of Current AI Detection Tools?
With the rise of AI Detector, all of us have been taking advantage of them but we also need to know about their limitations.

AI detection tools, such as AI Checkers and AI Detectors, have become essential in various fields, including education, content moderation, and cybersecurity. These tools aim to identify content generated by artificial intelligence, ensuring authenticity and accountability. However, despite their growing importance, current AI detection tools have several limitations that can impact their effectiveness. Understanding these challenges is crucial for improving detection technology and managing expectations.
1. Inability to Detect Advanced AI Outputs
As AI models become increasingly sophisticated, their outputs resemble human-generated content more closely. Advanced AI systems, such as large language models, produce nuanced, coherent, and contextually appropriate text that often bypasses detection algorithms. This arms race between AI generation and detection technologies makes it challenging for AI Detector to keep up.
2. High False Positive Rates
One significant limitation of AI Checkers is their tendency to produce false positives—instances where human-generated content is incorrectly flagged as AI-generated. This can lead to unfair consequences, particularly in educational or professional contexts, where originality is paramount. Such inaccuracies undermine trust in detection tools.
3. Limited Contextual Understanding
AI detection tools often rely on linguistic patterns and statistical analysis to identify AI-generated content. However, these tools lack deep contextual understanding. For example, they may struggle to differentiate between well-written human text and machine-generated text when both adhere to similar linguistic structures.
4. Over-Reliance on Training Data
AI Detectors are only as good as the data they are trained on. If the training data does not include examples of newer AI-generated content, the detection tool may fail to recognize such outputs. This dependency on up-to-date training data creates a lag in the tool’s effectiveness.
5. Difficulty with Multimodal Content
Many AI systems now generate multimodal content, combining text with images, audio, or video. Current AI Checkers and Detectors are often specialized for one type of content, such as text. This specialization limits their ability to detect AI-generated content across different modalities.
6. Ethical and Privacy Concerns
Some AI detection tools require extensive data collection to function effectively, raising ethical and privacy concerns. For instance, tools that analyze user-generated content may inadvertently collect sensitive information, leading to potential misuse or breaches of privacy.
7. Lack of Standardization
The field of AI detection lacks standardized benchmarks for evaluating tool performance. Without consistent metrics, it’s challenging to compare the effectiveness of different AI Checker and Detector or assess their reliability in real-world applications.
8. Computational Costs
AI detection tools often require significant computational resources to analyze content effectively. This can make them inaccessible to smaller organizations or individuals with limited resources, creating an uneven playing field in combating AI misuse.
Strategies to Address These Limitations
While current AI detection tools have limitations, ongoing research and innovation offer potential solutions:
-
Continuous Updates: Regularly updating AI detection algorithms with new data can help them adapt to evolving AI technologies.
-
Hybrid Approaches: Combining AI detection with human oversight can improve accuracy and reduce false positives.
-
Development of Multimodal Tools: Expanding detection capabilities to handle text, images, and other modalities will enhance their utility.
-
Ethical Design: Ensuring that AI detection tools prioritize user privacy and operate transparently can address ethical concerns.
Conclusion
AI detection tools are invaluable in maintaining authenticity and accountability in a world increasingly influenced by artificial intelligence. However, their current limitations highlight the need for continued development and refinement. By addressing challenges such as advanced AI outputs, false positives, and contextual understanding, researchers and developers can create more robust tools. Ultimately, balancing innovation with ethical considerations will be key to the future success of AI Checkers and Detectors.
What's Your Reaction?


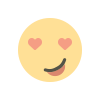



